Given an m x n
grid. Each cell of the grid has a sign pointing to the next cell you should visit if you are currently in this cell. The sign of grid[i][j]
can be:
1
which means go to the cell to the right. (i.e go fromgrid[i][j]
togrid[i][j + 1]
)2
which means go to the cell to the left. (i.e go fromgrid[i][j]
togrid[i][j - 1]
)3
which means go to the lower cell. (i.e go fromgrid[i][j]
togrid[i + 1][j]
)4
which means go to the upper cell. (i.e go fromgrid[i][j]
togrid[i - 1][j]
)
Notice that there could be some signs on the cells of the grid that point outside the grid.
You will initially start at the upper left cell (0, 0)
. A valid path in the grid is a path that starts from the upper left cell (0, 0)
and ends at the bottom-right cell (m - 1, n - 1)
following the signs on the grid. The valid path does not have to be the shortest.
You can modify the sign on a cell with cost = 1
. You can modify the sign on a cell one time only.
Return the minimum cost to make the grid have at least one valid path.
Example 1:
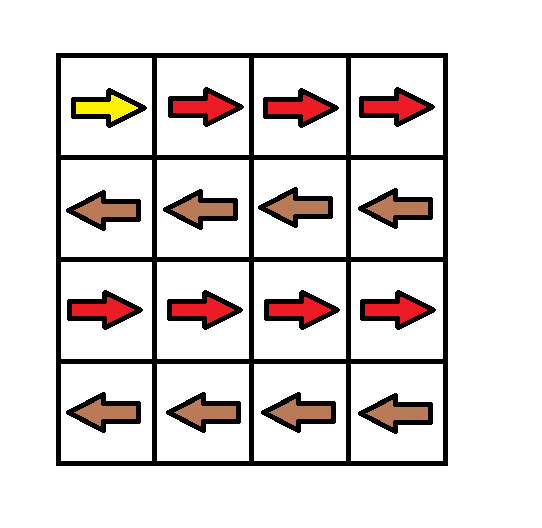
Input: grid = [[1,1,1,1],[2,2,2,2],[1,1,1,1],[2,2,2,2]] Output: 3 Explanation: You will start at point (0, 0). The path to (3, 3) is as follows. (0, 0) --> (0, 1) --> (0, 2) --> (0, 3) change the arrow to down with cost = 1 --> (1, 3) --> (1, 2) --> (1, 1) --> (1, 0) change the arrow to down with cost = 1 --> (2, 0) --> (2, 1) --> (2, 2) --> (2, 3) change the arrow to down with cost = 1 --> (3, 3) The total cost = 3.
Example 2:
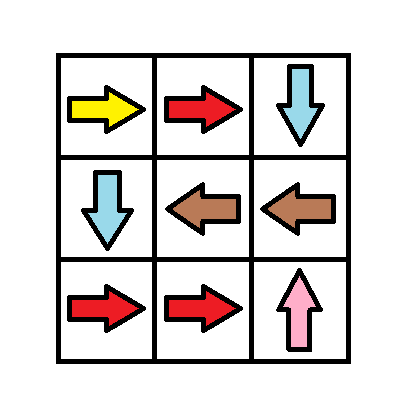
Input: grid = [[1,1,3],[3,2,2],[1,1,4]] Output: 0 Explanation: You can follow the path from (0, 0) to (2, 2).
Example 3:
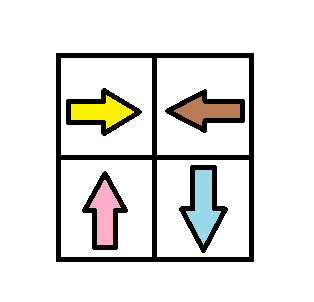
Input: grid = [[1,2],[4,3]] Output: 1
Constraints:
m == grid.length
n == grid[i].length
1 <= m, n <= 100
1 <= grid[i][j] <= 4
Problem Statement:
You are given an grid, where each cell contains a sign directing the next move. The signs are:
- 1: Move right.
- 2: Move left.
- 3: Move down.
- 4: Move up.
You start at the top-left cell (0, 0) and aim to reach the bottom-right cell . You can change the sign on any cell at a cost of 1. The goal is to determine the minimum cost to make at least one valid path from to .
Approach Explanation:
This problem can be tackled using a modified version of Dijkstra's algorithm. The key idea is to minimize the cost of traversing the grid to reach the destination. Here's the step-by-step approach:
1. Understanding Movement Costs:
- Moving in the direction indicated by the current cell's sign costs 0.
- Changing the direction to any other valid move costs 1.
2. Data Structures:
- Use a priority queue to always process the cell with the smallest cost first.
- Maintain a
cost
array to track the minimum cost required to reach each cell.
3. Algorithm:
-
Initialization:
- Start with the top-left cell with cost 0.
- Push this cell into the priority queue.
-
Process Cells:
- For each cell, explore all possible directions:
- If moving in the indicated direction:
- Add it to the queue with no additional cost.
- If moving in another direction:
- Add it to the queue with cost + 1.
- If moving in the indicated direction:
- For each cell, explore all possible directions:
-
Stop Early:
- If you reach the bottom-right cell , return the cost.
-
Terminate:
- The priority queue ensures cells with the smallest cost are processed first, guaranteeing the minimum cost to reach the destination.
Solution in C#:
using System;
using System.Collections.Generic;
public class Solution {
public int MinCost(int[][] grid) {
int m = grid.Length, n = grid[0].Length;
int[][] directions = new int[][] {
new int[] {0, 1}, // right
new int[] {0, -1}, // left
new int[] {1, 0}, // down
new int[] {-1, 0} // up
};
int[,] cost = new int[m, n];
for (int i = 0; i < m; i++)
for (int j = 0; j < n; j++)
cost[i, j] = int.MaxValue;
var pq = new PriorityQueue<(int row, int col, int cost), int>();
pq.Enqueue((0, 0, 0), 0);
cost[0, 0] = 0;
while (pq.Count > 0) {
var (x, y, c) = pq.Dequeue();
if (x == m - 1 && y == n - 1)
return c;
if (c > cost[x, y])
continue;
for (int k = 0; k < 4; k++) {
int nx = x + directions[k][0];
int ny = y + directions[k][1];
int newCost = c + (k + 1 == grid[x][y] ? 0 : 1);
if (nx >= 0 && nx < m && ny >= 0 && ny < n && newCost < cost[nx, ny]) {
cost[nx, ny] = newCost;
pq.Enqueue((nx, ny, newCost), newCost);
}
}
}
return -1; // Should not reach here
}
}
Example Walkthrough:
Input:
grid = [[1,1,1,1],
[2,2,2,2],
[1,1,1,1],
[2,2,2,2]]
Execution:
- Start at (0, 0) with cost = 0.
- Move along the indicated path:
- .
- Change the arrow at to go down .
- Continue:
- .
- Change arrows at and , costing an additional 2.
Output:
Cost = 3
Complexity Analysis:
- Time Complexity:
- : The priority queue processes each cell once, with logarithmic operations for insertion and deletion.
- Space Complexity:
- : For the cost array and the priority queue.
While the priority queue-based solution (modified Dijkstra's algorithm) is an optimal approach for this problem, I'll also include other potential approaches. Each method has its own advantages and trade-offs, and their inclusion provides a more comprehensive understanding of the problem-solving strategies.
Alternate Approaches:
1. Breadth-First Search (BFS) with a Deque:
- Idea: Instead of using a priority queue, use a double-ended queue (deque) to implement a 0-1 BFS.
- How it works:
- Push cells with a cost of 0 to the front of the deque.
- Push cells with a cost of 1 to the back of the deque.
- Advantages: This avoids the overhead of maintaining a heap (priority queue), making it faster in practice for grid-based problems.
- Complexity:
- Time: because each cell is processed at most once.
- Space: .
Implementation:
using System;
using System.Collections.Generic;
public class Solution {
public int MinCost(int[][] grid) {
int m = grid.Length, n = grid[0].Length;
int[][] directions = new int[][] {
new int[] {0, 1}, // right
new int[] {0, -1}, // left
new int[] {1, 0}, // down
new int[] {-1, 0} // up
};
int[,] cost = new int[m, n];
for (int i = 0; i < m; i++)
for (int j = 0; j < n; j++)
cost[i, j] = int.MaxValue;
var deque = new LinkedList<(int x, int y, int c)>();
deque.AddFirst((0, 0, 0));
cost[0, 0] = 0;
while (deque.Count > 0) {
var (x, y, c) = deque.First.Value;
deque.RemoveFirst();
if (c > cost[x, y]) continue;
for (int k = 0; k < 4; k++) {
int nx = x + directions[k][0];
int ny = y + directions[k][1];
int newCost = c + (k + 1 == grid[x][y] ? 0 : 1);
if (nx >= 0 && nx < m && ny >= 0 && ny < n && newCost < cost[nx, ny]) {
cost[nx, ny] = newCost;
if (k + 1 == grid[x][y])
deque.AddFirst((nx, ny, newCost));
else
deque.AddLast((nx, ny, newCost));
}
}
}
return cost[m - 1, n - 1];
}
}
2. Dynamic Programming (DP):
- Idea: Use a dynamic programming table
dp[i][j]
where each cell represents the minimum cost to reach that cell. - How it works:
- Start from the top-left cell .
- Iteratively update the minimum cost for each cell by checking all possible directions.
- Use multiple iterations until no further updates can be made.
- Advantages: Intuitive to implement and easy to reason about.
- Disadvantages: Less efficient for large grids, as it may require multiple iterations to propagate the cost values.
- Complexity:
- Time: , where is the number of iterations (can be up to in the worst case).
- Space: .
Implementation:
public class Solution {
public int MinCost(int[][] grid) {
int m = grid.Length, n = grid[0].Length;
int[][] directions = new int[][] {
new int[] {0, 1}, // right
new int[] {0, -1}, // left
new int[] {1, 0}, // down
new int[] {-1, 0} // up
};
int[,] dp = new int[m, n];
for (int i = 0; i < m; i++)
for (int j = 0; j < n; j++)
dp[i, j] = int.MaxValue;
dp[0, 0] = 0;
bool updated = true;
while (updated) {
updated = false;
for (int i = 0; i < m; i++) {
for (int j = 0; j < n; j++) {
for (int k = 0; k < 4; k++) {
int ni = i + directions[k][0];
int nj = j + directions[k][1];
if (ni >= 0 && ni < m && nj >= 0 && nj < n) {
int newCost = dp[i, j] + (grid[i][j] == k + 1 ? 0 : 1);
if (newCost < dp[ni, nj]) {
dp[ni, nj] = newCost;
updated = true;
}
}
}
}
}
}
return dp[m - 1, n - 1];
}
}
Comparison of Approaches:
Approach | Time Complexity | Space Complexity | Pros | Cons |
---|---|---|---|---|
Priority Queue (Dijkstra) | Optimal for weighted shortest path problems. | Overhead of maintaining a heap. | ||
BFS with Deque (0-1 BFS) | Faster for grid problems with unit costs. | Harder to understand than Dijkstra. | ||
Dynamic Programming (DP) | Intuitive and easy to implement. | Can be slower for large grids. |
Including multiple approaches not only provides flexibility in implementation but also helps understand different paradigms for solving grid-based pathfinding problems.